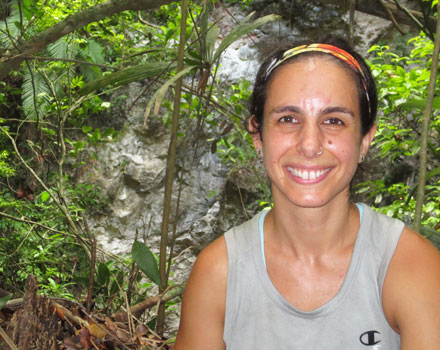
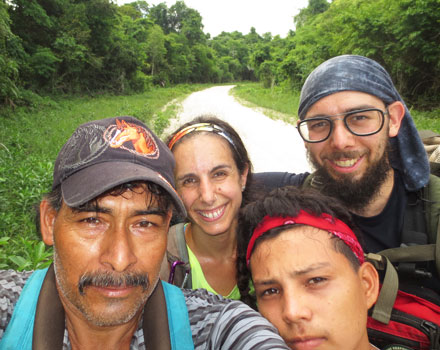
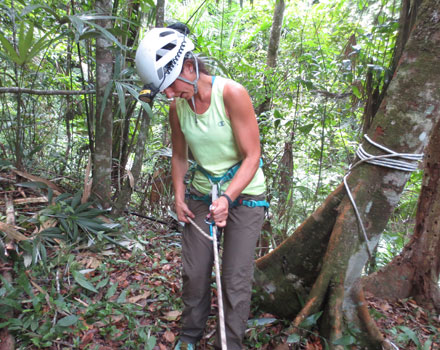
Leila Character was in Guatemala chopping through the tropical forest with a machete and trying to avoid the getting even more covered in bug bites than she already was. Character and her team were looking for caves at a Maya archaeological site with the hope of finding climate records. They would later correlate the climate to Mayan population demographics at the site.
“This sort of thing is fun, but also it's like miserable at the same time,” she recalls about the trip. The team mostly couldn’t find what they were looking for and she found herself wondering aloud if a computer could make the process more efficient. It was an epiphany that led to groundbreaking research using AI and a career in archaeological machine learning.
With an undergraduate degree and a master’s in geology – completed with a thesis on geoarchaeology – Character was always driven to explore nature. Her work experience included working as a geologist for an oil company and an environmental consulting company and as an environmental specialist for the State of Tennessee where she, among other things, implemented the first ever Tennessee State Parks recycling initiative (“Go Green with Us”) and organized community garden workshops across Tennessee in underserved communities and food deserts.
Machine learning in archaeology is a new area, and Character says there are about 10 people that consistently publish their work. Because of the lack of guidance, she felt concerned that she did not know what she was doing, but…
Now “Dr. Character,” she says continuing to earn a PhD was not in her plans; “a PhD is a commitment, she explained. “And if you don't find something that you're excited about, a PhD, doesn't help you make more money; it’s not necessarily a path to a better job.”
But, on that fateful trip to Guatemala, things “just changed on the dime” and she discovered a new direction.
After coming back from Guatemala to the University of Texas, Character investigated what she now calls “archaeological machine learning,” but couldn’t find anything similar to what she was envisioning. She learned researchers had used machine learning to detect sinkholes but not for small, horizontal cave entrances. Machine learning in archaeology is a new area, and Character says there are about 10 people that consistently publish their work. Because of the lack of guidance, she felt concerned that she did not know what she was doing, but…
“I just kind of dove in, and it's amazing what you can make happen when you just kind of ignore those feelings and keep chugging along,” Character says.
It took her more than a year, but she built a simple but powerful model called “random forest” that would help find those sites deep in the jungle.
“It had lots of exciting potential for finding caves and caves that might contain climate records as well as archaeological material,” she says.
But to validate the results of her model, Character had to go back to where it all started – Guatemala – the following summer. “I think it's important, if it's possible for the person building these models, to also know what these features look like in the field,” she explains. The process is called “ground-truthing” and the results would either make or break the model. It worked; her efforts paid off.
As Character was celebrating the first victory, she got a call from her advisor’s colleague asking to apply what she had learned with machine learning to a dataset of 17,000 Maya structures. As a result, she began using “deep learning,” a methodology that would help further train the model, seeing all the different ways that Mayan features might look across the landscape.
That’s when another adviser suggested she should apply to the Naval Research Enterprise Internship Program (NREIP) and Naval History and Heritage Command (NHHC) Underwater Archaeology Branch (UAB). As Character was applying for the NREIP internship, she did not have a clear idea what it would be. “I was like how could my skills possibly fit into anything that is naturally aligned with what I'm already working on,” she explains. But being an open-minded person, she went for it, as she did previously with the machine learning idea.
She adjusted the model she used for the Maya project for building the one to detect shipwrecks. Working with the Navy not only helped her to expand her knowledge and enhance her skills, but gave her an important opportunity to dive deep into the work she loved.
“I was fully focused on building this model which is what much of the rest of my dissertation moving into my career is built on,” she recalls. “That was tremendous, just the ability and the drive to fully focus on this for several months.” She also met many talented people that she is still working with.
Character says she now wants to create a single framework that can easily be adjusted so that those working in the field do not have to start from scratch. As the project develops, she hopes to go back to Guatemala and travel to Mexico to further validate the results, a trip that had been postponed by the pandemic.
And, she often thinks about all those different futures and alternative timelines that she might have had, if not for the trip to Guatemala. An avid rock climber, she coaches at the local rock-climbing gym.
“If I hadn't stayed for the PhD, I would've done something else I love and that probably would've had to do with climbing.”